Image Annotation for Machine Learning: Unlocking the Future of AI
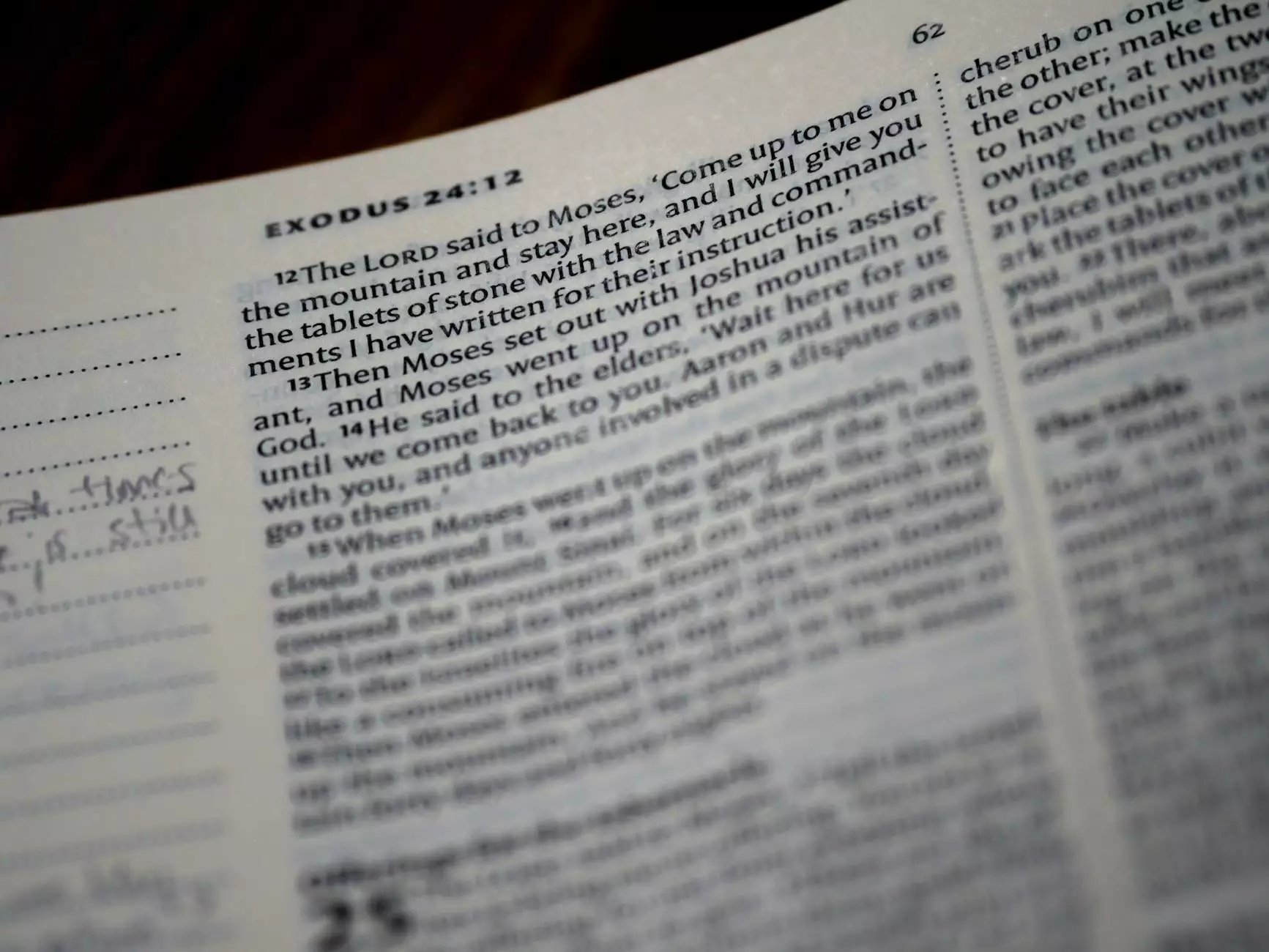
The rise of artificial intelligence (AI) and machine learning (ML) has ushered in a new era where data is the driving force behind innovation and efficiency. Among the many components that facilitate effective machine learning, image annotation stands out as a crucial aspect. Whether you are developing applications for autonomous vehicles, security systems, or medical imaging, the quality of your image data and its annotations can significantly impact the performance of machine learning models.
What is Image Annotation?
Image annotation involves the process of labeling images so that a machine learning model can recognize and learn from them. This process can include identifying objects, tagging relevant features, and providing contextual information that aids in the understanding of the image. The importance of accurate annotations cannot be overstated; they directly affect the model's ability to learn and make predictions.
Why is Image Annotation Essential for Machine Learning?
In the realm of machine learning, especially in computer vision, annotated images are the foundational building blocks. Without precise annotations, models trained on this data may yield poor results, leading to inefficiencies and inaccuracies. Here are some reasons why image annotation for machine learning is essential:
- Training Data Quality: High-quality annotations ensure that the training dataset is a reliable source for teaching AI. Inaccurate labels can lead to skewed outcomes.
- Improved Accuracy: Well-annotated datasets help improve the accuracy of machine learning models, resulting in better performance when applied to real-world scenarios.
- Adaptability: Proper annotations allow models to adapt to new data and environments, a vital characteristic for systems like self-driving cars.
- Scalability: Structured annotations make it easier to scale machine learning solutions across different domains or applications.
Types of Image Annotation
There are various methods for annotating images, and each method serves specific machine learning objectives. Here are some common types of image annotation for machine learning:
1. Bounding Box Annotation
This is one of the most basic forms of image annotation where a rectangular box is drawn around the object of interest. This method is particularly effective for object detection tasks, for example, identifying vehicles in traffic or animals in wildlife photography.
2. Semantic Segmentation
Unlike bounding box annotation that labels the entire object as a single unit, semantic segmentation involves labeling each pixel of an object individually. This provides finer detail and is especially useful for tasks that require understanding the shape and contours of objects, such as in medical imaging or landscape analysis.
3. Instance Segmentation
This technique builds upon semantic segmentation by distinguishing between separate instances of the same object class. For instance, in an image with multiple dogs, instance segmentation can differentiate between each individual dog, providing a more accurate dataset for training.
4. Keypoint Annotation
Keypoint annotation focuses on marking specific points of interest on an object. This method is commonly used in pose detection tasks where the position of joints in human figures needs to be predicted.
5. Polygon Annotation
In cases where objects are irregularly shaped, polygon annotation is used. This method provides a more precise outline of the object than a simple bounding box, making it ideal for applications such as satellite imaging or vegetation mapping.
Choosing the Right Data Annotation Tool
Given the critical role of image annotation for machine learning, choosing the right tools is paramount. The wrong choice can lead to inefficiencies, inaccuracies, and increased costs. Here are some factors to consider when selecting a data annotation tool:
1. Ease of Use
The tool should have an intuitive interface that allows annotators to work efficiently without extensive training. Look for a solution that provides straightforward navigation and helpful resources.
2. Scalability
As your project grows, your data annotation needs will likely expand. Choose a tool that can scale with your operations, accommodating larger datasets and more complex annotation tasks.
3. Support for Various Annotation Types
Make sure the tool supports multiple annotation methodologies suitable for your specific machine learning needs. This flexibility can be crucial as your projects evolve.
4. Collaboration Features
For larger teams, collaboration features are essential. Look for a platform that allows multiple users to work on the project simultaneously and facilitates easy communication and tracking of changes.
5. Quality Control Mechanisms
Ensuring the quality of annotations is essential. Select a tool that provides mechanisms for quality assurance, such as review workflows and feedback loops.
Image Annotation Platforms: A Deep Dive
With many tools available in the market, it can be overwhelming to choose the right one. Here's how KeyLabs.ai stands out in the realm of data annotation platforms:
1. Comprehensive Annotation Options
KeyLabs.ai offers a wide variety of annotation services to cater to different needs, ranging from bounding box annotation to advanced semantic segmentation and instance segmentation. This full-service approach allows businesses to tailor the data preparation process according to their machine learning requirements.
2. AI-Powered Automation
To enhance productivity and save time, KeyLabs.ai incorporates AI-powered features that suggest annotations based on prior labeled data. This automation accelerates the annotation process while maintaining high accuracy rates.
3. Real-Time Collaboration
The platform is built for teams. KeyLabs.ai offers real-time collaboration tools so that project members can annotate, review, and provide feedback concurrently, creating a dynamic and efficient workflow.
4. Customizability and Flexibility
KeyLabs.ai allows you to customize your annotation preferences. This flexibility ensures that you can adapt the platform to meet your unique needs without being constrained by predefined workflows.
5. Robust Support Systems
KeyLabs.ai prides itself on maintaining strong customer support. Their team is readily available to assist with any issues or queries, ensuring that your annotation projects run smoothly.
The Future of Image Annotation in Machine Learning
As machine learning continues to evolve, so does the necessity for proficient and accurate annotations. Innovations such as image annotation for machine learning will play a pivotal role in the AI landscape. We can expect advancements in annotation technologies, including machine learning algorithms that can automate the annotation process even further. In addition, the integration of augmented reality (AR) and virtual reality (VR) technologies could redefine how data is collected and annotated.
Moreover, as more industries embrace AI, we will likely see a surge in demand for specialized annotation services that cater to specific fields such as healthcare, agriculture, and autonomous vehicles. The need for precise, high-quality annotations will become even more pronounced, reinforcing the importance of choosing the right data annotation tool and platform.
Conclusion
In summary, image annotation for machine learning is a fundamental aspect in unlocking the capabilities of artificial intelligence. The demand for high-quality annotated data is set to rise as organizations increasingly rely on machine learning for various applications. Platforms like KeyLabs.ai provide robust solutions tailored to meet these needs, incorporating advanced technology and user-centric features.
The future is bright for those who invest in quality image annotation. Whether you are a startup or a large enterprise, leveraging the right tools and practices can position you ahead in the ever-competitive landscape of machine learning and AI development.
For more information about our data annotation tools and platforms, visit KeyLabs.ai.