Unraveling the Power of Data Labelling Services in Software Development
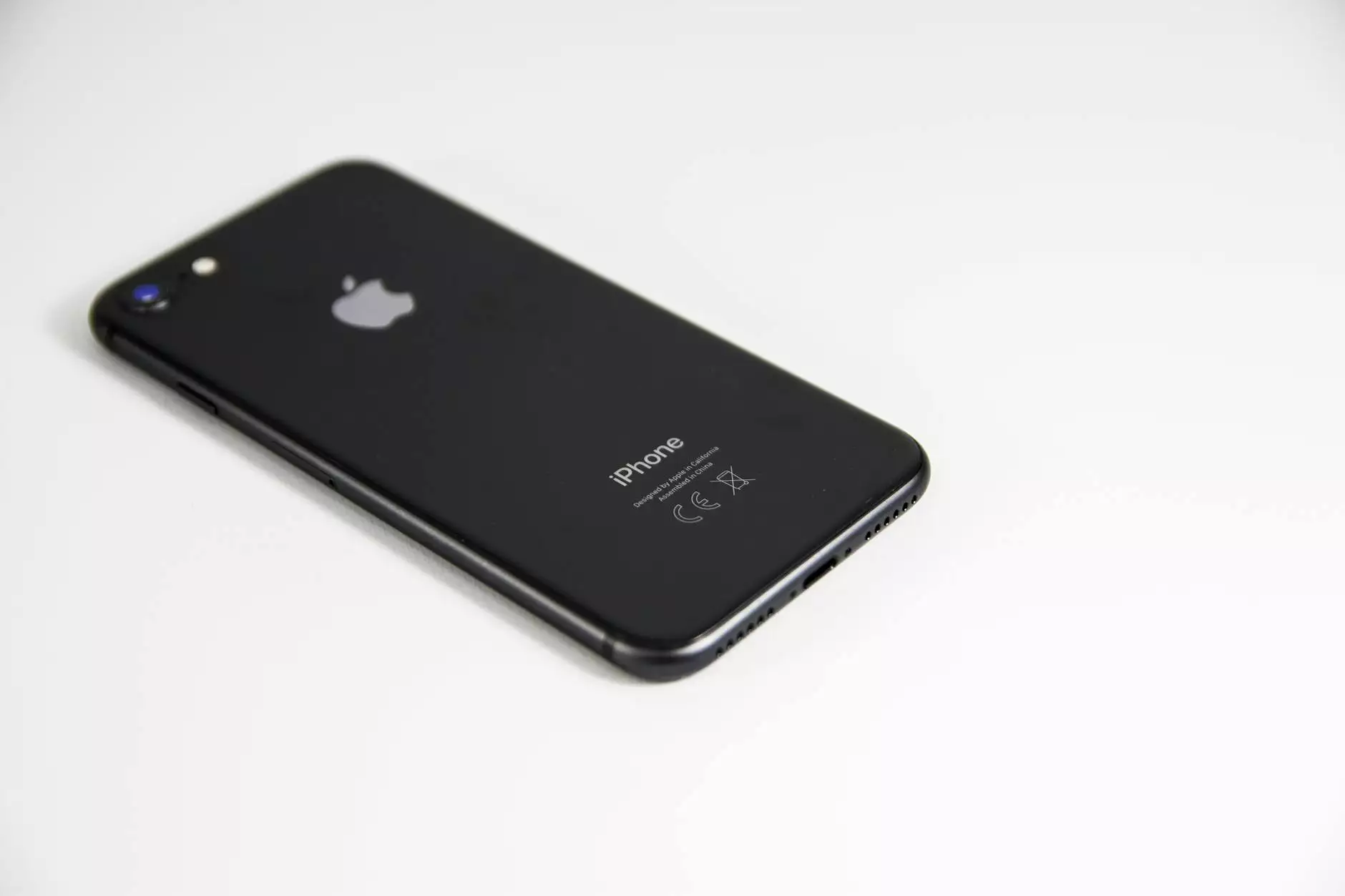
The landscape of software development is constantly evolving, and at the heart of this innovation lies the crucial practice of data labelling services. As businesses strive to harness the power of artificial intelligence (AI) and machine learning (ML), the need for accurately labelled data becomes not just advantageous, but essential.
Understanding Data Labelling Services
Data labelling is the process of annotating or tagging data, turning raw data into structured formats that machines can understand. This service is particularly vital in training machine learning models, as the accuracy of these models depends heavily on the quality of the labelled data provided. Through meticulous labelling, data becomes a valuable asset that can drive informed decision-making and innovation in various software applications.
The Importance of Accurate Data Labelling
Why is accurate data labelling crucial? The answer lies in the functionality of AI systems. Here are some reasons that highlight the importance:
- Precision in Machine Learning: Inaccurately labelled data can lead to significant errors in model training. For instance, in image recognition, if an image of a cat is mislabelled as a dog, the model may learn incorrect patterns, affecting its future predictions.
- Enhanced Decision Making: Businesses rely on ML-driven insights to facilitate decision-making. High-quality labelled data ensures that these insights are based on reliable models, supporting better strategies and outcomes.
- Competitive Advantage: Organizations that invest in robust data labelling can leverage AI tools faster and more effectively, positioning themselves ahead of competitors who may overlook the significance of quality data.
Types of Data Labelling Services
Data labelling services encompass various methods and techniques tailored to different types of data:
- Image Labelling: Involves annotating images with tags or bounding boxes to identify objects within the images. Useful in sectors like healthcare (for identifying anomalies in scans) or automotive (for training autonomous vehicles).
- Text Labelling: This includes categorizing text documents or classifying sentiments. Applications range from email filtering systems to sentiment analysis tools.
- Audio Labelling: Involves tagging audio datasets for applications like voice recognition and sound classification. It is crucial for developing AI systems that understand and respond to human speech.
- Video Labelling: Annotating video data for various applications, including surveillance systems and action recognition, enhances the ability of AI to interpret dynamic environments.
How Data Labelling Services Work
The Labelling Process
The data labelling process typically involves several steps to ensure quality:
- Data Collection: Gathering raw data from various sources relevant to the project.
- Annotation Guidelines Development: Creating clear guidelines and protocols for labelling to ensure consistency and accuracy.
- Labelling: Trained annotators manually label the data or use automated tools to assist in the process, applying the guidelines established.
- Quality Assurance: Implementing a rigorous review process to verify the accuracy of the labels, including spot checks and audits.
- Final Review and Delivery: The labelled data is finalized and delivered to the clients in the specified format, ready for integration into their machine learning models.
Benefits of Outsourcing Data Labelling Services
Outsourcing data labelling services can be a game changer for businesses looking to enhance their software development processes:
- Cost Efficiency: Hiring in-house teams for data labelling can be expensive. Outsourcing allows companies to tap into skilled professionals at a fraction of the cost.
- Access to Expertise: Specialized data labelling service providers offer a wealth of experience and expertise in various labelling techniques, ensuring high-quality outcomes.
- Scalability: As your data needs grow, it's easy to scale up or down the services provided, allowing businesses to remain flexible in their operations.
- Focus on Core Competencies: Outsourcing enables companies to focus on their primary business functions while leaving the data labelling process to the experts.
Choosing the Right Data Labelling Service Provider
Selecting a data labelling service provider is a critical decision that can impact your project’s success. Here are key factors to consider:
- Experience: Look for providers with a proven track record in your industry and relevant experience in various data types.
- Technology Utilization: The best providers leverage technology to streamline labelling processes while maintaining quality, such as using AI to assist human annotators.
- Quality Assurance Practices: Ensure the provider has robust quality assurance measures in place to minimize errors and improve the reliability of the labelled data.
- Transparency and Communication: A good provider should maintain clear communication, keeping clients informed about the process and progress of their data labelling.
Common Use Cases of Data Labelling Services
Data labelling services are employed across various sectors. Here are notable examples:
Healthcare
In the healthcare sector, data labelling services enable the development of advanced diagnostic tools by annotating medical images, electronic health records, and various health data. Accurate labels improve the efficiency of disease detection and patient management systems.
Automotive
The automotive industry relies on data labelling for enhancing autonomous driving technology. By accurately labelling road signs, pedestrians, and other vehicles in video data, companies develop more reliable self-driving algorithms.
E-commerce
E-commerce platforms use data labelling services for product classification and image recognition to optimize search functionality and improve customer experiences.
Future Trends in Data Labelling Services
The field of data labelling is continuously advancing. Here are some trends to watch:
- AI-Assisted Labelling: Leveraging AI tools in the labelling process will reduce the time required for annotation while maintaining high accuracy.
- Increased Demand for Multimodal Data Labelling: As businesses adopt AI systems that integrate multiple data types (text, image, audio), the need for comprehensive data labelling services will grow.
- Remote Work Opportunities: The trend of remote work has opened up access to a global talent pool for data annotators, enhancing the quality and diversity of labelling services.
Conclusion
In conclusion, the significance of data labelling services in the realm of software development cannot be overstated. They serve as the backbone for training accurate machine learning models, ultimately driving innovation and efficiency in various industries. By investing in quality data labelling, businesses can elevate their operations and stay competitive in an increasingly data-driven world.
For companies looking to gain a foothold in AI and machine learning, partnering with a reputable provider of data labelling services, such as Keymakr, may well be one of the most strategic decisions they can make.